by Mohadeseh Ganji
A few months ago, I presented on how AI is enabling transformation in the energy industry at the Chief Data Analytics Officer conference in Melbourne and the AI Summit for Business in Sydney. This topic has generated keen interest, so I have written this article to share the key points with a broader audience.
Application of advanced analytics is not new in the energy sector, examples include demand and generation forecasting, network health and voltage compliance monitoring, intelligent automation, and loss of neutral detection in order to avoid shock hazards.
I would like to focus on the trends by which AI is going to empower the transformation of the energy industry, namely, cost efficiency, customer centricity and distributed energy resources (DER) management.
Cost efficiency
A portion of every electricity bill is associated with moving energy and maintaining the huge network of electricity assets.
Most electricity is generated at power stations and carried long distances at high voltage by the electricity transmission network, then the lower voltage electricity distribution network brings electricity to consumers. Electricity network owners in Australia are responsible for operating and maintaining this large network of assets to ensure reliable, safe energy is available for consumers; industrial, residential, small and large scale. These networks include thousands of kilometres of transmission and distribution lines.
The scale, labour-requirement and risk involved in maintaining and operating such networks, means that even slight efficiency improvements translate to huge savings which contribute to more affordable energy for everyone and provide funding for investment in network solutions which will enable distributed energy resources, such as rooftop solar, to flourish.
There are a variety of examples that AI is contributing in cost efficiencies, I am going to mention a couple of examples.
Part of maintaining electricity networks is a need to create a digital model of the network, sometimes referred as “digital twin”. The capture of these assets can be completed using aerial and ground-based photography or LiDAR technology. The volume of data being captured by these processes is enormous and yet doesn’t add much value unless processed, classified and geospatially mapped as a model. The ability to utilise AI to automatically detect the network asset within this data is of significant value. Using machine learning and deep learning, network assets such as poles, cross-arms, insulators and conductors can be automatically detected and classified with accuracy equivalent to or even better than a human. Furthermore, AI is also enabling automatic detection of defects on assets and their severity.
As another example, consider vegetation monitoring and management, a fundamental requirement of network management, as trees and other plants impose risk of fire and damage if in contact with wires and other assets. In vegetation management, for which LiDAR technology is often used, AI is augmenting rule-based systems not only to detect network assets but also buildings, roads and vegetation, with higher accuracy.
Customer centricity
Consumer behaviour is changing in the digital world, customers are becoming prosumers, proactively wanting to take control over their energy finances, and environmental impact.
Customers are exposed to massive information in the world of digitisation, and they are influenced by many sources including online social networks and media.
Customers are contributing significantly to shaping the future landscape of energy. Australia is again one of the world leaders with 20% solar penetration among residents. How does AI contribute to the increase in solar penetration? As an example, enabled by AI and other advanced technologies, the google sunroof project brings solar potential analysis to customers in just a few clicks. It considers the location, roof angle, sun radiation, cloud movement patterns and vegetation nearby, and quantifies the useful sun exposure and potential savings, then even offers a financing options for customers – all in one place. The ease of gaining customised information and potential saving accelerates the decision-making process and enables faster penetration of solar.
Personalised communication and offerings cannot scale without the help of AI. We all know one size doesn’t fit all. Everyone has different energy needs, social and behavioural drivers, and financial preferences. Most energy retailers and energy management solution providers are using AI to understand natural segments among their customers and predict which offering would suit their need. They then communicate these personalised offers at scale and instantaneously.
Tech start-ups in the energy industry are increasingly growing. Enabled by AI and advanced analytics they are innovating in various areas including bringing electricity to our home cheaper, more sustainably, more efficiently, and in a more transparent manner. They are engaging with us, making it easy, and empowering us to make decisions which are right for us as individuals.
Distributed Energy Resources
In the past we had the luxury of building coal fired power stations without having to pay the full price of their impact – we then realised climate change is real, its impacts are fast increasing and that we must do something about it. As a result, we are facing a rapid decarbonisation of energy sector, meaning less coal power stations and more solar, wind, and potentially Hydrogen. We are a lucky country with abundant solar and wind resources. According to the Clean Energy Regulator, we are installing renewables at the rate of 250W per person, per year. This is equivalent to a couple of major coal fuelled power stations being built every year. If we continue this rapid uptake of renewables in next decade, it is estimated that we will reach 50% renewables by 2024 and fast approach 100% renewable by 2030. This means the electricity sector must be transformed rapidly to respond to this rapid disruption.
Imagine a world where most buildings have solar panels and battery storage working together using ‘smart devices’ to provide monitoring and control. It is early days for electric vehicle adoption but in one-to-two decades, forecasts show more than 50% of cars will be electric. These all add additional complexity to the way the electricity ecosystem operates, making it more difficult to predict supply and demand and maintain the network. Research has shown concepts of sharing and social connectedness is appealing to customers when it comes to energy, making them more willing to participate in energy sharing and trading scenarios.
In the future, more and more customers will have energy monitoring and analytics devices not only giving them real-time insight into their energy usage but also providing options to earn money or share and even donate energy. Decisions will need to be made on when to charge or discharge a battery, export surplus energy to the grid, charge vehicle, control air conditioners, participate in demand response programs, and so on. The list goes on for the choices available to energy users at any point in time
Even more decisions must be made in real time by network and market operators with additional complexity of dynamic demand, supply and network constraints.
Both rapid penetration of renewables and the growing needs and power of customers come with great challenges and big opportunities. What exactly that optimal balance in the dynamic fast-changing energy ecosystem will look like is unknown. What is clear is that AI is not going to be nice-to-have but rather a must-have which impacts every aspect of the future energy landscape.
Are we ready for the future? Are we even pedalling hard enough to keep up with the rate of disruption? Now is the time to ask these questions and think carefully about how these changes will impact your organisation.
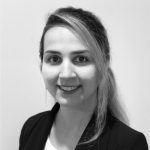
Moha is a PhD qualified AI and Advanced Analytics practice lead. She is experienced in driving value through advanced analytics and helping organisations adopt AI and advance technologies in different industry sectors such as finance, retail and energy.
Moha has taught in graduate programs and delivered several workshops and industry speeches at conferences such as Smart Energy Systems conference, Chief Data Office, Machine Learning and AI summit and All Energy Australia in 2018 and 2019. Moha was listed among top 25 analytics leaders Australia and ranked 5th among the top leaders by IAPA (Institute of Analytics Professionals Australia). Moha is passionate about Advanced technologies, renewables and sustainability.